Artificial Intelligence (AI) should be used to augment, not replace, human creativity and intelligence.
The term, coined in 1955 by John McCarthy, was defined by him as “the science and engineering of making intelligent machines.” Historically, AI techniques like machine learning have been used to make recommendations and help us better understand information.
AI is at the core of our Student Impact Platform and has been since the company’s founding in 2011. We use AI in predictive modeling, a type of supervised machine learning, to predict student outcomes and help institutions create and carry out effective student success initiatives.
Today, advances in AI, like generative AI used in platforms such as ChatGPT, build on this concept by using AI technologies like large language models (LLMs) to create original content. This advancement in AI technology opens doors to endless possibilities and raises important questions about AI’s societal impacts and governance.
Understanding the application of AI and its impact across your technology stack is an important step in responsibly navigating this new frontier. In this article, I share how Civitas Learning has incorporated AI technologies in its platform for over a decade and how we approach leveraging evolving technologies within our solutions.
How the Civitas Learning Student Impact Platform Applies AI
Our founders started Civitas Learning to translate higher education’s vast student data into actionable insights that institutions need to help their students succeed. For over a decade, we’ve pioneered and iterated upon our market-leading AI-based data analytics platform to develop comprehensive student success software that drives positive student outcomes across the entire student lifecycle.
AI is at the core of our Student Impact Platform and has been since the company’s founding in 2011. We use AI in predictive modeling, a type of supervised machine learning, to predict student outcomes and help institutions create and carry out effective student success initiatives. Supervised machine learning trains algorithms to recognize patterns by using carefully selected data. Once trained, these algorithms can analyze fresh data to forecast future student outcomes.
One of the algorithms we frequently use is called Random Forests, a type of machine learning algorithm that works by building many decision trees and then averaging their predictions. Random Forests are highly accurate and easy to interpret, making them a good choice for student predictions. However, we regularly research additional algorithms to ensure the highest quality in our predictions.
UTSA Achieves 16% Retention Lift with Data-Activated Student Support Ecosystem
How AI-Based Predictive Modeling Helps Institutions Improve Student Success
Predictive modeling provides student success leaders and their teams with a prediction or signal pointing to what might happen without intervention. Far from being deterministic, a prediction is a machine-learning-aided call to action that empowers student success professionals to help students improve that outcome.
Predictions are projections, not certainties. They should be used to inform timely, no-harm interventions and high-quality outreach. It’s important to balance the intelligence provided by predictive analytics with the expertise of human professionals. Instead of relying solely on the predictions provided by the analytics system, responsible use entails using insights as input for decision-making and creative problem-solving.
Several key predictions drive Civitas Learning’s student insights: Persistence Prediction — “How likely are you to retain this student?”, Completion Prediction — “How likely is this student to graduate on time?” and Initiative Analysis —” Which student success initiatives work?”
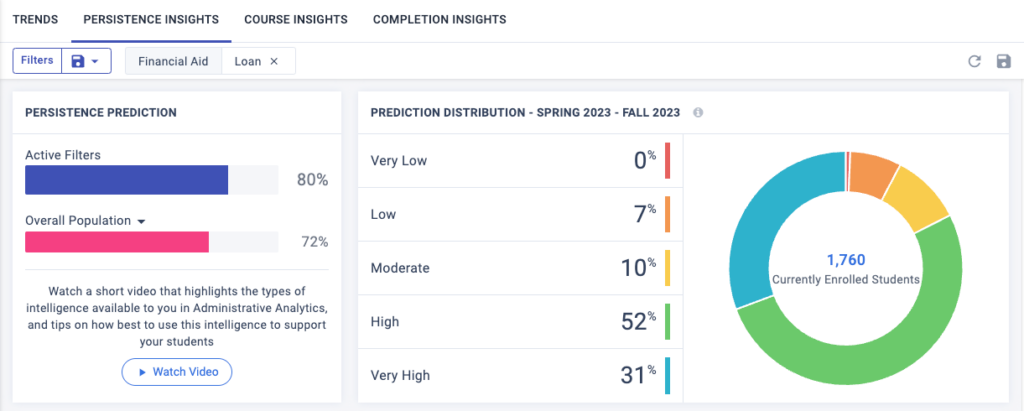
Each student is scored on their likelihood to persist on Day 1 of a term and then every week thereafter (every day in our Advising Analytics and Workflow solution). Scores range from 0% to 100%. Once a term is over, the scores from Day 1 can be analyzed against what happened to evaluate the model, the results of which inform adjustments to be made when the model is retrained.
These scores are far more predictive of persistence than conventional methods used by institutions, such as watching to see who falls on academic probation. On day one, this prediction score was found to identify 82% of the students who later failed to persist, and accuracy continues to improve with additional training throughout the term.
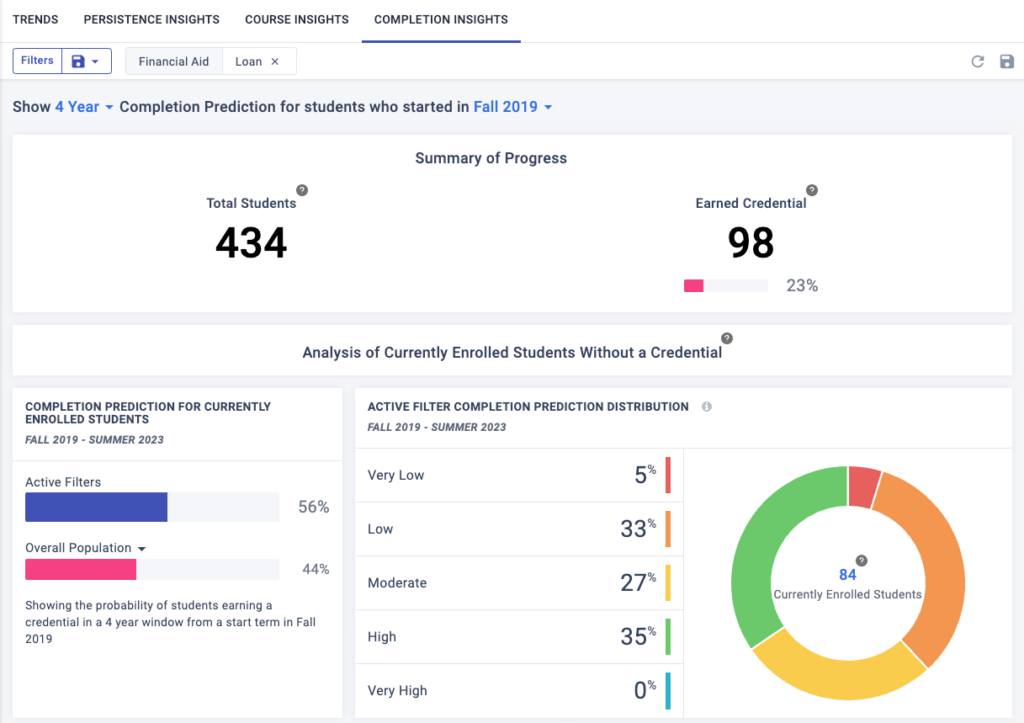
Just like persistence predictions (which predict retention into the next term), completion predictions are built from your institution’s data and reflect the unique student experience on your campus, using the same data modeling and machine learning platform. Each student gets an individual completion prediction based on their performance and activity relative to the historical trends for who completes degrees at your institution.
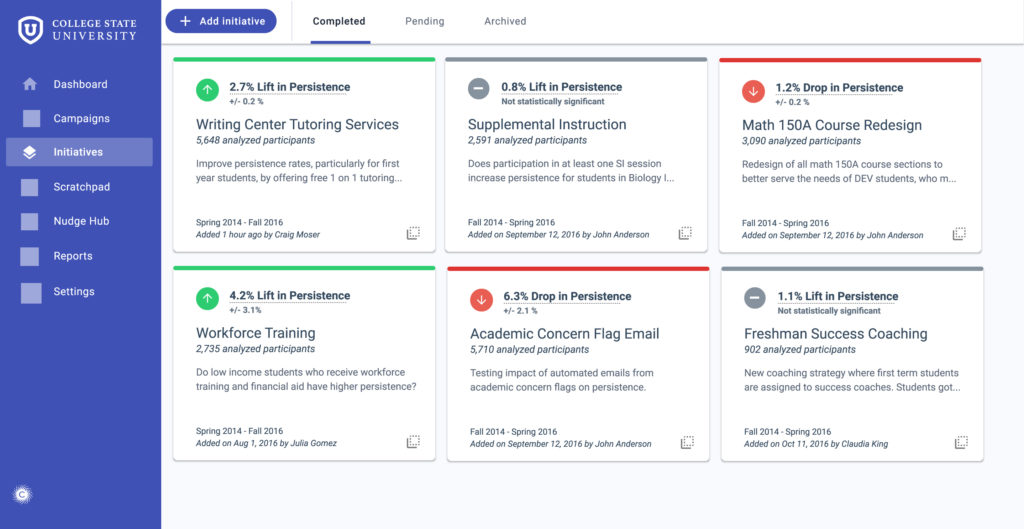
The Student Impact Platform also includes a tool for Initiative Analysis, which helps institutions quickly analyze student success programs’ effectiveness. Each initiative is measured for its effectiveness using a Prediction-based Propensity Score Matching (PPSM) technique that melds traditional statistical methods with predictive machine learning for a robust and precise evaluation.
4 Guiding Principles for Using Student Success Analytics
How AI-Based Predictive Modeling Works in the Student Impact Platform
Typically, 100s of variables are considered for modeling. Most of these model predictors are new variables based on your institution’s basic data. We use your data to build out derived variables, which surface time-dependent and comparative information like sections withdrawn or LMS activity relative to peers that help reveal which students need attention.
Ultimately, the most powerful and important predictors are our derived variables in combination with student engagement information because they are based on changeable behaviors rather than fixed demographics. Additionally, the configuration of each institutional model is not set in stone. Your institution can test or add additional model variables through custom configuration work.
What’s Next?
Responsibly using AI technologies to help higher education institutions improve equitable student outcomes is at the core of our mission. It’s been woven through our work from the founding of Civitas Learning over a decade ago.
At Civitas Learning, we believe deeply in our mission to provide technology, services, and support to empower partners to help every student succeed. As AI technologies evolve, we are actively researching opportunities to apply the benefits of new technologies to our suite of solutions.
Our latest research centers around ways to use AI technologies to help leaders better understand course demand. We’re working to help institutions better leverage their resources by offering the courses students need when they need them. Course Demand Analytics provides leaders with actionable insights they need to monitor real-time course enrollment to address issues like low and high fill rates and demand forecasting to predict short and long-term course needs accurately.
Have questions or want to discuss how we can support your work to improve student success? Don’t hesitate to get in touch with us.